library(data.table)
library(ggplot2)
set.seed(2)
<- data.table(
d_obs x = sort(runif(100, 0, 2*pi))
):= sin(x)]
d_obs[, eta := rpois(.N, 200)]
d_obs[, n := rbinom(.N, n, plogis(eta))]
d_obs[, y
# we only observe 30% of the data generated
:= rbinom(.N, 1, 0.7)] d_obs[, y_mis
Random walk priors
First order random walk
For regular spacings, a first-order random walk prior can be specified as:
\[ \begin{aligned} \eta_0 &\sim \text{Logistic}(0,1) \\ \delta &\sim \text{Normal}(0, 1) \\ \sigma_\delta &\sim \text{Exponential}(1) \\ \eta_{[1]} &= \eta_0 \\ \eta_{[k]} &= \sum_{i = 2}^{N}(\eta_{[k-1]} + \delta \sigma_\delta) \\ \end{aligned} \]
Simulate data from an oscillator:
Naive implementation of a first order random walk in stan.
data {
int N;
// the way the model is set up it does not matter if some of the n's are
// zero because the likelihood uses y_sub, which is obtained by reference
// to the missing indicator y_mis, which explicitly says that there were
// no observations at the given value of x.
array[N] int y;
array[N] int n;
vector[N] x;
array[N] int y_mis;
int prior_only;
// priors
real r_nu;
} transformed data {
// x_diff gives us the variable spacing in x and allows us to scale
// the variance appropriately
vector[N-1] x_diff;
// the number of observations we truly had once missingness is accounted for
int N_sub = N - sum(y_mis);
// our truly observed responses (successes) and trials
array[N_sub] int y_sub;
array[N_sub] int n_sub;
//
for(i in 1:(N-1)){x_diff[i] = x[i+1] - x[i];}
// go through the data that was passed in and build the data on which
// we will fit the model
int j = 1;
for(i in 1:N){
if(y_mis[i] == 0){
y_sub[j] = y[i];
n_sub[j] = n[i];1;
j +=
}
}
}parameters{
// the first response
real b0;
// offsets
vector[N-1] delta;
// how variable the response is
real<lower=0> nu;
} transformed parameters{
// the complete modelled mean response
vector[N] e;
// this is the variance scaled for the distance between each x
// note this is truly a variance and not an sd
vector[N-1] tau;
//
vector[N_sub] eta_sub;
// adjust the variance for the distance b/w doses
// note that nu is squared to turn it into variance
for(i in 2:N){tau[i-1] = x_diff[i-1]*pow(nu, 2);}
// resp is random walk with missingness filled in due to the
// dependency in the prior
1] = b0;
e[// each subsequent observation has a mean equal to the previous one
// plus some normal deviation with mean zero and variance calibrated for
// the distance between subsequent observations.
for(i in 2:N){e[i] = e[i-1] + delta[i-1] * sqrt(tau[i-1]);}
// eta_sub is what gets passed to the likelihood
{ int k = 1;
for(i in 1:N){
if(y_mis[i] == 0){
eta_sub[k] = e[i];1;
k +=
}
}
}
} model{
// prior on initial response
target += logistic_lpdf(b0 | 0, 1);
// prior on sd
target += exponential_lpdf(nu | r_nu);
// standard normal prior on the offsets
target += normal_lpdf(delta | 0, 1);
if(!prior_only){target += binomial_logit_lpmf(y_sub | n_sub, eta_sub);}
} generated quantities{
// predicted values at each value of x
vector[N] p;
vector[N-1] e_diff;
vector[N-1] e_grad;
// compute diffs
for(i in 1:(N-1)){e_diff[i] = e[i+1] - e[i];}
e_grad = e_diff ./ x_diff;
p = inv_logit(e); }
<- cmdstanr::cmdstan_model("stan/random-walk-01.stan")
m1
= list(
ld N = nrow(d_obs),
y = d_obs[, y],
n = d_obs[, n],
x = d_obs[, x],
y_mis = d_obs[, y_mis],
prior_only = F,
r_nu = 3
)
<- m1$sample(
f1 iter_warmup = 1000, iter_sampling = 2000,
ld, parallel_chains = 1, chains = 1, refresh = 0, show_exceptions = F,
max_treedepth = 10)
Running MCMC with 1 chain...
Chain 1 finished in 3.0 seconds.
$summary(variables = c("nu")) f1
# A tibble: 1 × 10
variable mean median sd mad q5 q95 rhat ess_bulk ess_tail
<chr> <dbl> <dbl> <dbl> <dbl> <dbl> <dbl> <dbl> <dbl> <dbl>
1 nu 0.533 0.523 0.100 0.0962 0.390 0.710 1.00 1298. 1384.
Representation of output.
<- data.table(f1$draws(variables = "p", format = "matrix"))
d_out
<- melt(d_out, measure.vars = names(d_out))
d_fig <- d_fig[, .(
d_fig mu = mean(value),
q_025 = quantile(value, prob = 0.025),
q_975 = quantile(value, prob = 0.975)
= variable]
), keyby := gsub("p[", "", variable, fixed = T)]
d_fig[, ix := as.numeric(gsub("]", "", ix, fixed = T))]
d_fig[, ix := d_obs[ix, x]]
d_fig[, x
ggplot(d_obs, aes(x = x, y = plogis(eta))) +
geom_line(lty = 1) +
geom_point(data = d_obs[y_mis == 0],
aes(x = x, y = y/n), size = 0.7) +
geom_point(data = d_obs[y_mis == 1],
aes(x = x, y = y/n), size = 0.7, pch = 2) +
geom_ribbon(data = d_fig,
aes(x = x, ymin = q_025, ymax = q_975),
inherit.aes = F, fill = 2, alpha = 0.3) +
geom_line(data = d_fig,
aes(x = x, y = mu), col = 2) +
geom_point(data = d_fig,
aes(x = x, y = mu), col = 2, size = 0.6) +
scale_x_continuous("x") +
scale_y_continuous("Probability") +
theme_bw()
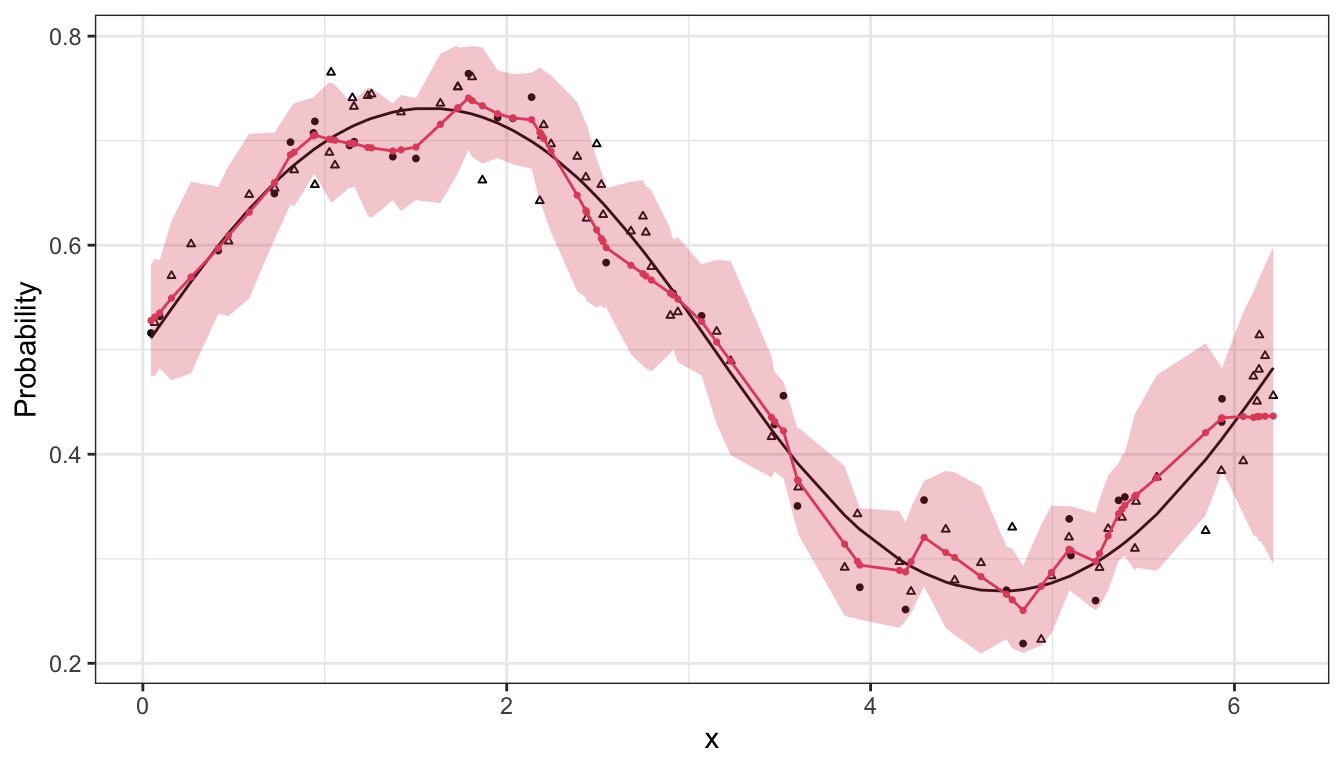
Second order random walk
The second order random walk for regular locations has density
\[ \begin{aligned} \pi(x) \propto \exp\left( -\frac{1}{2} \sum_{i=2}^{n-1} (x_{i-1} - 2x_i + x_{i+1})^2 \right) \end{aligned} \]
The main term can be interpreted as an estimate of the second order derivative of a continuous time function. But this is not generally suitable for irregular spacings of x [1].